Use and limitations of AI in support of OSINT
- 0.5
- 1
- 1.25
- 1.5
- 1.75
- 2
Audio: Welcome to The World of Intelligence, a podcast for you to discover the latest analysis of global military and security trends within the open source defense intelligence community. Now onto the episode with your host, Harry Kemsley.
Harry Kemsley: Hello and welcome to this episode of World of Intelligence at Janes. I'm Harry Kemsley, your host as usual and as usual, I have my co- host, Sean Corbett. Hello Sean.
Sean Corbett: Hi Harry. Good to be here as ever.
Harry Kemsley: As ever. Good to see you Sean. So, Sean, I can't remember the number, but I'm very confident that there have been very few podcast episodes we've done where we haven't touched on the use, the problems with et cetera with artificial intelligence. I think we've used the letters AI numerous times and I think it's about time that we actually took that topic on by itself. So today I am delighted to invite an expert in artificial intelligence. Keith Dear. Hello, Keith.
Keith Dear: Hello, Harry.
Harry Kemsley: Keith Dear is managing director of Fujitsu's Center for Cognitive and Advanced Technologies. He previously served as an expert advisor to the UK Prime Minister on Defense Modernization and the Integrated Review. A former 18- year intelligence officer for the Royal Air Force, he's also served in numerous operational campaigns. He holds a doctorate in experimental psychology from the University of Oxford, an MA in terrorism and counterterrorism from King's College, London and is also a fellow at the defense and security think Tank RUSI. So Keith, let's get started by making sure that we're all on the same page about what we mean by artificial intelligence. Perhaps you could do us privilege of just giving us your understanding of what we mean by artificial intelligence to get us started.
Keith Dear: So definitions of AI are contested everywhere, like all interesting things, right?
Harry Kemsley: Sure.
Keith Dear: And we used to work on terrorism and counter terrorism. We still don't have a singular good definition of what that is. AI is really similar. If you get the brief potted history of AI, you have the cybernetics movement that came out of World War II and Norbert Wiener. Interestingly, as an aside for a military audience, one of Norbert Wiener's ways into understanding what he called cybernetics was through working on anti- aircraft artillery that would be automatically slewed, so you had a feedback loop of a sensor that would sense something which slewed the anti- aircraft artillery and then would shoot it down. And he was like, " Well, this is more effective than people at doing this." If we have that feedback loop of sensing something and then you have a processing in the middle that makes sense of the information coming in and then that directs an action in the real world, well, that cybernetic loop is common to humans, it's common to animals. And he wrote a book, a famous book called Cybernetics in Human and the Machine, which describes all this. And then in 1956, one of the founding fathers of the modern movement of AI, John McCarthy came up with the term artificial intelligence to rebrand cybernetics. Why did he do it? Well, for all of the best reasons in academia, because he wanted funding and artificial intelligence was much more compelling than cybernetics. So then you have the emergent field of AI, which really is just a continuation of the cybernetics movement but was beginning to think less in symbolic terms and more in what we understand today as machine learning. So the idea that you could build models that sense adapted and responded and learned from the data, it wasn't that you encoded a kind of recipe or a menu of human intelligence. So AI in that sense, can be divided neatly into two fields. There's symbolic AI, which it most famous through the Garry Kasparov example, when IBM's Deep Blue beat Garry Kasparov at chess in 1997. So that's symbolic AI. You can think of it as a recipe, a kind of if then else and encoding of everything humans know, lots of different games of chess than it wins. Incredibly complex, can deliver superhuman performance, but is in the end limited by what we know.
Harry Kemsley: Yep.
Keith Dear: Then you have the field of machine learning, which is the one that's in the news every day since about 2010. That's the canonical example is AlphaGo Zero beating Ke Jie at the game Go that learns from data. What does that give you? Well, it learns from data, it spots patterns we wouldn't have spotted, and it gives you superhuman performance, but it lacks explainability and crudely put, because there are variations on the underlying algorithms, but it's that kind of neural networks that is the modern machine learning that we talk about when we're talking about large language models or any of the breakthroughs in the last decade. So where does that leave? What is AI? The reason this is such a contested field is we continuously redefine it. I think about the best definition that most of us end up settling on is it ends up being all of those things that, those things that otherwise would've required a human to do and then we end up leaving, well what is intelligence? Well, it's all those things that machines can't yet do and there's an increasingly narrow range of those. So we keep redefining what we mean by intelligence according to how we define artificial intelligence. So it's another way of saying all that is, is it's an essentially contested concept, but hopefully that gives you some idea of what it is we're talking about.
Harry Kemsley: Absolutely. Sean?
Sean Corbett: Yeah, I was just going to say as ever, Keith, a PhD level of something that I would probably give the Luddites version of. But as you say, I think the only consistent thing about definitions of AI is the fact that there is no consistent definition of AI.
Keith Dear: Yeah.
Sean Corbett: But I've just been looking at the DOD's definitions at the moment actually, and even they're not settled actually, but they do try and make something that is actually applicable because they've got to keep it down to basic levels. And I think as you said, their definition that I like is" Computer systems designed to replicate a range of human functions and continuously get better at assigned tasks." So for me, picking out the specific activities and against this, an applied task to do things that humans could do and of course we can talk about the future next that humans can't do.
Harry Kemsley: Okay, all right, well look, if we agree then that the definition is constantly evolving, and by the way, I think that's probably appropriate because technology's constantly evolving. Therefore what it can do and how you define it probably needs to evolve with it. What I want to try and pull us towards then is, okay, so we've defined the almost undefinable, what is AI? Although we've had a great insight to the history of it and what that might mean for us today. Let's move on then to, so where is it being used in the intelligence community? Now in the recent past, Keith, we've had various contributors and guests on talking about things like deepfakes and how AI is supporting that and indeed counter deepfake AI technology. We've talked about how it can be used in the relatively dull but important aspects of collect and collate for example, and how it finds for example, again, sentiment and so on. These are the sorts of things that we've heard about. But how would you summarize the uses of AI looking at it through the prism of national security and the technologies being used in that sort of context?
Keith Dear: So I mean the neat summary comes from, I guess, in some ways, the center that I now run. It's the Center for Cognitive Technologies and the reason it's cognitive technologies is there's any technology that draws insight or foresight from data. There's a whole raft of psychologists out there, Seligman and Baumeister being two of the prominent ones who would describe humans as Homo Prospectus by which it means we're not wise humans, but rather we are humans that anticipate the future and plan for it. That sounds a lot like what we all did in the intelligence world and what the OSINT world does today. So anything that draws insight or foresight from data. So what that immediately tells you is that the cognitive technologies, which includes, and is centrally is AI, cover the full range of the intelligence cycle. And there are multiple reports out there that can give you an idea of different elements it can do and I think, I mean we're interested in how you might apply it, for example in ISTAR Tasking to make sure that you've got the right asset in the right place at the right time. That seems to me a fundamentally symbolic problem, which is another way of describing symbolic systems as an expert system. So if you can encode what a practitioner can do, does in a kind of linear way, i. e., if this, then that else x, then you can encode it into symbolic AI. If it's a thing where the human is searching in vast data sets, well what machine learning can already do is search in a kind of mathematical breadth or a breadth of different information sources beyond anything that a human can begin to do and equally can think in a recursive depth beyond anything that a human can do. So I know that you know that I know therefore I should, and we can get up to roughly six or seven levels of that. There is no practical limit on how far a machine can do that and that's why we talk in the center about cognitive technologies revolutionizing decision making. So that gives you the high level kind of academic answer to your question. In practical terms, the gap between what AI and ML and the way we're talking about it can do and what it is actually doing in the intelligence world is vast. And I suspect that we'll come on to some of the barriers to adoption, but I mean if it's insight or foresight from data, I think it would be a bit of a fools errand for me to try to say well it can do this or it can do this, it can do this, because we'll be here for another three days.
Harry Kemsley: Yes.
Keith Dear: If it's cognitive, it's about decision making. There are decisions at every stage of the intelligence cycle. That's where you apply AI and machine learning.
Harry Kemsley: Where do you think, Sean, I'll come to you in just a second. Where do you think it is currently the most effective and where do you see concerns about the effectiveness of the use of AI in that intelligence cycle you talked about?
Keith Dear: So I think where we are applying, again, depending on the tyranny of definitions, but where it is being applied most at the moment is in the analysis of vast quantities of image data.
Harry Kemsley: Okay.
Keith Dear: And I think that there is movement towards trying to do that for full motion video capture where the number of hours add up something like seven years, last time I looked at this and that was like 2015, of full motion video footage captured from various assets that you would know only too well. I think that computer vision element is, it's well understood that humans have been completely overwhelmed by the amount of data we need to search.
Harry Kemsley: Sure.
Keith Dear: The process by which our imagery analysts undertake is broadly a linear function and equally that having models that can just search vast amounts of data and spot things we wouldn't have spotted is incredibly useful. So I think it's principally in that element of, I suppose, you described that as the processing of information, right, before you have to go and say, " Well what does this mean? What should we do?"
Harry Kemsley: Yeah.
Keith Dear: I think overwhelmingly that's where the applications are focused because we know we've long been, since been defeated by the size of the data sets we're searching in. I think in OSINT you're beginning to see the application of similar algorithms but being used to predict information in things like financial data in sort of vast online data sources. And you see it being increasingly applied for what you might think of kind of financial crime, but which ends up, you know, you see the kind of investigations Bellingcat does ends up being just as relevant to national security. So again, in theory, everywhere in practice focused on computer vision and on vast data sets that have long since defeated us or that we didn't previously exploit.
Harry Kemsley: Yeah, we'll come back to why it might not have been spread elsewhere in terms of its use in just a moment. But Sean, I know you wanted to step in at that point.
Sean Corbett: Yeah, I think Keith's really hit the main point for me and your starting point that I know that your two big things, the insight and the foresight is key. In terms of where we need to go and want to go with artificial intelligence, is the so what that I always known, talked about, it's doing that extra, what does this mean?
Harry Kemsley: Yeah.
Sean Corbett: And that's why the definitions do really matter for our world because you've mentioned one end of the spectrum, which at the moment is what I think most people understand as AI is that it's taking vast quantities of data that the human being just simply cannot absorb and do anything with and managing them in an effective way, collecting, collating, managing them in an effective way that the human then can do that. So that's one end of the spectrum, but where I would like to get to obviously, ultimately is the ability to support that insight and foresight. And the last thing that you said that was really important is to assist decision making. It was interesting what you said about machine learning, because I mean there's another debate, probably a pub debate over is machine learning a subset of AI or is it separate? And I know there are different views on that and I've had a few arguments about that one as well. But I think the machine learning element is quite important because when you are talking about huge amounts of data and I think about the now historical little green men all over eastern Ukraine that were really high, in fact impossible to actually identify on the vast quantities of imagery. But if as long as you've got the right training data and you train the algorithms correctly, the AI, if you want to call it that, can actually spot these things.
Harry Kemsley: Yeah.
Sean Corbett: Far quicker, far more efficiently than the human being.
Keith Dear: Well I think that's right. I think the other kind of, forgive me for asking a question in response to the question, but I think it's also what timeline are we talking about for what AI can do? If it was 2010 and we were having this conversation, DeepMind was what? I think it had four employees and no products and there was no AI anywhere outside the lab. Now look where we are and every major breakthrough between 2012 and 2018 has come with increases in computational power through the application of machine learning. And I think it's easy to lose track of just how far and fast that has gone. So there's been a 300, 000 times increase in computational power between 2012 and 2018 alone. If that's your mobile phone battery, it would now last eight years on a single charge. So it gives you the idea of just how far this has gone and there's a guy called Rich Sutton who writes and thinks about this, he describes it as the bitter lesson in which we imagine that in order to match human performance, we're going to need these really complex expert systems that we spend hours working out how do Harry and Sean analyze this problem and then we encode it in this really complex code. And because we are so smart, it has to be incredibly complex and what we've actually seen is just applying massive amounts of compute to very large data sets and adding more parameters over time is enough to give us superhuman performance in multiple fields. And what we're now seeing is phase shifts. So you get linear improvements in the performance of models with increases in computational power, but you also get these things that computer scientists are terrified of calling emergence because intelligence in the human brain is emergent. Nevertheless, I think there's a reasonable case to be made, I don't want to wander in too deep in that controversy, but there is a reasonable case to be said that this is emergence, but you get phase shifts. So things that you could not have predicted the model would do and then you add computational power and suddenly it can, so an example would be, in ChatGPT, suddenly it couldn't do anything in Persian. Suddenly it could do accurate Persian question and answering and we didn't think it would be able to do that previously. It couldn't do arithmetic, suddenly it could do arithmetic, it couldn't translate intelligence processing automation, suddenly it could do it. And so these phase shifts are... There's one of the reasons we definitely shouldn't get into the existential threat debate because it's another like podcast in itself, but the reason people are worried is because of these emerging capabilities or phase shifts. Why should we care in the OSINT world? Well because we're saying well what will it be able to do in 10 years? Well we've already seen things that in 2010 you would've been laughed at for claiming that machine learning could do just through these massive increases in computational power and now we're seeing these phase shifts of it suddenly being able to do things and I think from a national security perspective that is worrying, but if we stick to what can it do, it's also really exciting.
Harry Kemsley: Yeah.
Keith Dear: I mean it begins to imply like spotting our little green men, well maybe it can spot them much, much earlier than we can in data sets that we would never have thought to look at as long as you can tell it what to optimize against. And of course that's a fundamental challenge, but as long as you can tell it what you want the model to optimize against is, I think we will see that superhuman performance on the ever increasing amount of data that we see available throughout open source information.
Harry Kemsley: Keith, do you think those phase shifts, as you described, are they accelerating? Is the time between a phase shift shortening as the system, is it, let's not go into the emergent piece as you say, because that is another podcast, but is the rate of change, the rate of improvement actually accelerating?
Keith Dear: So the answer to that question is yes. The corollary to it is how long can that continue for and that is another super contentious debate. So it was a paper in December last year from the large language model company AI generative AI company, Anthropic, a rival to OpenAI that they published where, if I remember, there were eight examples they gave of phase shifts of things that models, they allow a large language model couldn't do and then suddenly could, and that is a relatively new phenomena and increases in computational power well we're expecting the speed and cost of training our AI models to drop, what was I told the other day? I think it's 16 fold in the autumn with the release of the next generation of processors by a commercial rival who I'm not going to name. So if you look at that, I think we can expect to continue to see an increase in those kind of phase shifts. But I mean we're in an era here of radical uncertainty as well and so that seems likely, but I mean it would be, again, a bit of a false errand to say this is precisely what's going to happen, which because we just don't know.
Harry Kemsley: So I mean for the audience of which I'm a member by the way, that doesn't really understand how AI does what it does and the phase shift acceleration, is there a reason to be concerned about that? Is there a reason for us to be worried that we are quote" not in control," and out of... we're out of control with what AI is capable of doing and learning to do?
Keith Dear: So that is such a fraught debate. My view is if you're thinking on the longer time scale, yes. I think the... If you imagine intelligence to be a hierarchy at which, and I don't think this is absolute, but which broadly humans are at the top of and there's an argument I read once and I've been misattributing ever since, but the reason the tiger in the cage isn't because its weapons aren't better than ours, it's not because it's not stronger than ours, it's because it's not smarter than us. So do we just end up being the...? I think there's the philosophical and important argument there. The problem with that argument is it breaks down with its collision with practical reality because people say, " Right, we need a pause on AI research." Well what the hell are you pausing? Are you banning statistics? Are you going to ban-
Harry Kemsley: It's out of the box, yeah it's out of the box.
Keith Dear: ... the element of biology?At best you might delay it but then you're delaying it whilst others accelerate and that's probably not where we want to be. So should we be worried? Probably, yes. But yeah.
Harry Kemsley: My question, Keith, is more in the context of within intelligence we talk about the assurance of the data and the intelligence we're working with for decision support.
Keith Dear: Yep.
Harry Kemsley: And we seek to trust the process and Sean, I'm going to come to you on this, because I know it's a matter that you hold close to your heart. We seek to trust it because we understand it. We can trust it and therefore we can get a level of assurance in the data and intelligence. If it's running quote" into a black box that's accelerating away from us," then the ability to trust and be assured by it degrades, in a human sense. Sean, I'm going to let you step in here because I know this is definitely something you want to talk about, the human on and in the loop, but go ahead.
Sean Corbett: Yeah, and this is the conundrum that the intelligence analyst and obviously the decision makers are grappling with right now. To what extent do you trust the AI, a basic term I know, but do you trust the AI to actually do the cognitive stuff that then helps you to make the decision? Now for the analyst who, and we've talked about this many times, in fact we did a podcast on it, Tradecraft is king and you have to be able to show you're working and that's never going to change because somebody has to be accountable for this stuff.
Harry Kemsley: Well never's a strong word. I think and we say never.
Sean Corbett: Okay.
Harry Kemsley: I think what we heard from Keith is there is a time which we could foresee in which Tradecraft might actually go into a black box marked AI, but anyway, sorry to interrupt.
Sean Corbett: I say never because, you know, within the intelligence community, we're still looking at Tradecraft stuff that, and Neil Wiley, my good friend on last, would say, no, Tradecraft does evolve and I agree with that, but we are still absolutely driven by the policies that say, if you've come up with analysis, you need to tell us where you got that analysis from. And if you can't do that, and this is the cognitive bit that is really tricky, and just to finish off my first point was, I think that the amount of trust depends on what you're going to do, the results of that artificial intelligence with. If you're talking about kinetic activity or something like that where, you know, you do the algorithms that comes out with drop that bomb on that target, you have to have someone in the loop, in my view, that says, right, okay, taking everything into consideration and the international humanitarian law and law of armed conflict that says it has to be proportional, it has to be distinctive, et cetera, et cetera. There is a judgment call there in terms of, okay, is that the concrete military advantage strong enough that you can take a certain amount of risk? And that is where I think it gets difficult for people to say, " Okay, right, we've got really good algorithms, we can let that make the decision for us." That is different, I think, personally, from using algorithms that actually help you make a judgment in terms of supporting decision making. You could, because the comeback of that of course is well depends on what the decision making is, but this, for me, is the crux of the discussion that I think that there's certainly the intelligence community and other communities I think are struggling with.
Harry Kemsley: So let me send to that Keith, because I think that's a really, really key point. So as I said, trust in the data, in the decision support, giving a level of assurance for decision making, Sean's asserted that Tradecraft will need to develop. We had a conversation about that with an eminent Tradecraft expert in recent times. Where does AI sit in this discussion about Tradecraft trust, decision support and insurer assurance? How do you feel about the introduction of black box if that's what it is?
Keith Dear: So there's a huge amount here. I think it's should be... I've been looking forward to this part of the discussion. Look, I think firstly, before you can have explainable AI, you first have to have explainable humans. I think all of us have worked for many senior commanders for whom their decisions are quite black box and if you force them to rigorously interrogate, okay, could you please give me the logic on which that was based, the premises that lead to the deduction that led you to give that order, what data is that based on? The answers you get, shall we say, might be less than robust. I think if you look at the science, Tetlock's work on super forecasting, we know that the forecasters who are the most confident are also the most likely to be believed and also the most likely to be wrong. So the people that we trust the most and that have, I hesitate to say this realizing present company as I start down this road, but the people who have progressed in that system, I mean I did okay as well, you've got to look at yourself and think, well did I progress because of my confidence or my forecasting accuracy? Was I really that good at insight and foresight or was I just really good at convincing people to listen to me? Well, and there's verbal fluency is another thing that correlates with your chances of being believed but doesn't correlate with your chances of being right. So there are so many limits to human forecasting and the way humans make decisions. I mean another example which we said we might come to, so confabulation is an example, right? Confabulation is a term that comes out the neuroscience and psychology literature where there's a guy called Michael Gazzaniga and he ran experiments on split brain patients. So these are people who have epilepsy, they have huge, too much interconnectivity between the left and right side of the brain. So they cut the corpus callosum so the left and right brain can't communicate anymore and what that means is that one side of the brain is receiving one set of inputs and it can't communicate with the other side. So the point here is that without going all through the detail of the experiments is that he found that if he presented to one side of the brain an image that the other side of the brain couldn't see, well the left side has the bit that does our voice, it does the thinking, it does all of what we're doing now and it would make up completely plausible stories to connect what the right brain was indicating pointing at with the hand for example. So it would make up these hugely convincing stories to cover gaps in things that it did not know and he called that confabulation. The fact that we make up plausible sounding stories to cover gaps in our knowledge. What do we have with large language models now? Well when it doesn't know something, it confabulates. So there are a whole range. I could go through all of the different kind of psychological biases, illusion of explanatory depth. We think we understand things until people say, do you really understand it? And then you discover that actually the detailed workings are the thing you thought you understood you don't. Humans have loads of limitations that we don't discuss. I think that really matters in a practical way in relation to the conversation we've just had.
Harry Kemsley: Yeah, if I may, Keith, what I think you're saying is we need to understand just how flawed we are as decision makers, as humans, and that therefore accusations of flaws in AI which are potentially more predictable in terms of what they do and how they do it is actually a lesser of the two evils. Is that a fair summary?
Keith Dear: It's a fair summary and if you'll allow me to bring it back down to earth.
Harry Kemsley: Yeah.
Keith Dear: If you talk about, for example, the requirement to discriminate, to positively identify your target. Suppose, and this is not really a hypothetical example, but you could deploy a computer vision system inside, for example, an armored vehicle that would automatically find an enemy competently. It would look for the things that a soldier would look for, I can't remember all the S's, but there was shape, shadow and those things and you're like, okay, there's that. There's this person and maybe they have to be in uniform or maybe not because it's like, so you've got all of the parameters that you set beforehand in your rules of engagement and delegated authorities and such like, and your model can't always tell you precisely why it's decided that thing there is a target and that thing there is not, but nor can your soldier and particularly not under huge amounts of pressure in the battlefield. So all that really matters under those circumstances and I think this is just as true for deliberate targeting as time sensitive targeting, all that really matters is does the model have a lower false positive and false negative rate than the human? Does it decide that the target is a military target accurately when it is or less frequently than, does it make errors in that judgment less frequently than a human and in the inverse, does it mistakenly identify less frequently than a human? If it has that lower false positive and false negative rate, my argument for a long time has been we have a moral obligation to delegate authority to machines under those circumstances. Not only do we have a moral obligation, but because things only really change in defense under kind of legal pressure or the pressure of defeat in war. I think we're as likely to end up in court because the mother of a civilian has who has been killed or a soldier's mother, when the soldier has been killed for not making a decision when the model would've made, if you delegate authority, that person would not have died. I think we're as likely to end up in a court case sued for not having delegated authority to a machine that demonstrably had a lower false positive and false negative rate than we are for delegating it.
Harry Kemsley: Yeah, a fascinating, fascinating conversation this. I'm remembered, as you're describing it, a situation in another part of the world, hot and sandy, where a young man with a toy gun in his hand ran at a patrol. By the way the weapon, the weapon, the toy was made of metal, so if there's any sort of detection of metal, he was running towards a checkpoint with what appeared to be a weapon. The soldier on point decided to walk up to this young man, cuff him around the ear and send him on his way explaining as best he could in broken Arabic that that was actually a bad idea. What you're saying to me is that's a decision that could have been made by a machine with enough appropriate computational power I think is what you're saying and that the false positives around that kind of circumstance, and I will come to you in just a second, Sean, I can see you are leaning forward to get in, are a moral obligation that we should be moving to a place where we can reduce the number of errors in these ethical situations. Fascinating. Sean?
Sean Corbett: Well firstly I was just about to agree entirely with Keith, which will surprise him in no end. But this is another really key part of this for the open source intelligence, well any intelligence perspective actually is that can you trust the human more than the machine or vice versa? And I do agree with you, Keith, actually. There are times when actually people are not as, got to be careful what I say there, but not as logical perhaps as the machine would be. Now again, a little bit of a dit with no names or pack drills, but Keith and I were both experienced, we were both intelligence, senior intelligence specialists for a specific commander in theater who, because of his worldview, refused to look at our assessments, which were pretty aligned not just with ourselves but the community in terms of a campaign assessment. And he practically forbid us to actually brief anything on that saying, " Yeah, my military judgment says this." Now that is all sorts of conscious and unconscious bias, but it was based on our cognitive approach with lots and lots of reports that we were then assimilating ourselves. I just wonder, and I was thinking about this before the podcast is, had he been given a definitive assessment from an AI model, would he have been any worse or any better in terms of doing that? Well I think in this case the ego was all there and it didn't matter what evidence was in front of him. But what that got me to think is that to what extent are the senior decision makers or any decision makers going to be biased either in favor of or against AI models? And that is something that the community is definitely going to have to embrace. And of course the answer is a combination of both.
Keith Dear: No, I mean really I was going to agree in turn, I'm not sure in that particular individual it would've made much of a dent and I think that that begins to highlight the adoption challenges. One of the things I wrote in, way back in 2018 on AI models was that it would drive rigor into decision making, the application of AI machine learning. So there's an article for Air Power Review actually, one of the things it would do is drive rigor into decision making by forcing us to be explicit in our premises, our logic, the evidence and data one which we're making decisions. What I think I misunderstood and perhaps this is what maturity brings, is the problem with that is it holds up a mirror to humans that most of us don't want to look in. And when you take that and not just in defense to any senior decision maker and you start talking about the flaws and how they make decisions, I think most people know it, in truth. I think most people know when you really push. But the last thing anybody wants to do is acknowledge openly how flawed current senior decision making is in multiple different domains. And so I think it's a massive adoption challenge.
Harry Kemsley: You're unraveling the imposter syndrome that we all suffer from and work within a mask increasingly well as we get more mature. Let me just pivot this conversation just slightly to something that we've discussed, Sean, in the past about the adoption of open source as a source of decision support. And we've said many times, Sean, have we not, that there are still far too many parts of the intelligence community that are not using the abundant potential of open source. Let me pivot that conversation or the principle of that conversation, Keith, to you, in terms of, so given the moral obligation, given the potential lower false positives et cetera, why do we think AI is not yet more fully embraced in intelligence in government perhaps, but certainly in intelligence, given the nature of this podcast?
Keith Dear: See, I think there's a whole range of reasons. I mean, one of the things I've said perhaps slightly controversially but hopefully fun is AI's a bit like teenage sex. Everybody is talking about it. Everybody thinks everybody else is doing it. Very few people are actually doing anything. And so I think look, one problem is there aren't a whole load of models where you go, okay, I'm going to go to this industry, see how they're doing it and copy it, maybe in finance, in areas of finance. But do you know what? One of the things that... That was what I thought, but as I get closer and cleaner, what I do now is not just national security focused. Adoption in finance and banking is much lower than you might expect too. I think the barriers to adoption, one of them we've already talked about, you have to hold up a mirror to how decisions are made today and that leads to an awful lot of resistance. One is an instinctive thing, it kind of assumption about the superiority of human decision making, which I think is increasingly being exposed as flawed. One is a really important consideration, which is how little we actually understand human intelligence. It's kind of counter all the arguments I'm making. We know an awful lot of facts about the brain. We do not know how the brain works.
Harry Kemsley: Right.
Keith Dear: So a fundamental problem is not really understanding the process of human cognition in sufficient detail to be able to say, okay, this is the thing that humans do and therefore we can be completely confident we can delegate that with full authority. And not withstanding what I said before. So I think there's still an uncertainty factor. And then there are barriers in defense. One of the barriers has long been the need to label data, which increasingly large language models are doing faster and more accurately than humans can. Then you've got the ongoing challenge of, ICT programs are always the first thing to be cut in budgets, and so that slows down. There are many different things and I think there's something interesting there, which we may come onto if it's of interest, but I think around the politics of information, which I think is really important to delaying adoption. But I'll leave that as a, if it's of interest.
Harry Kemsley: That's a inaudible that one. So given that time is always against us, I'm going to just pull it across to a slightly different perspective if I may. We've mentioned in this podcast, and Sean, you and I have discussed in specific detail in the past, ethical considerations, risk evaluations. I mentioned earlier the trust and assurance aspects of it, which suggests that we are being careful in our adoption of the AI capabilities. Potential adversaries may be less risk averse and should that be a concern to us, should we be looking at what AI could be used for and thinking about that in terms of mitigating risks that might be emerging from potential adversaries? Keith, let me start with you on that.
Keith Dear: So yes, I think if China, in particular, has been talking about intelligent- sized warfare at least as early as 2011, so moving from informationized warfare to intelligent- sized warfare and just the fact that you've set that kind of clear aspiration as early as that suggests that the adoption, the invention and so forth that is necessary to get you there has been going on for a while, while we have been busily saying, " No, no, we'll never delegate authority to machines. There's got to be a human in the loop." We have all these concerns, we need to spend a load of time on AI ethics before we worry about adoption. So I think the ability of those systems to set a clear destination. I think also the information in the end is power. And when you have such a centralized system as the CCP has, it's much easier to demand that everybody shares their information with a one centralized authority that then allows you to train models at scale. That's really hard in departments is kind of what I was getting to the politics of it. If you're sat in the Home Office, you don't necessarily want to make all of your data available to the MOD because it's probably going to be used against you in the great Game of Thrones that is Whitehall, right? That obviously goes on in other countries, but a really heavily centralized system doesn't have that same distribution of power and therefore can centralize the data and information required to train models. And we have some evidence, it's very difficult to track in detail, but that there have been significant investments, particularly in China. I think, I wrote in 2018 that Russia was talking the talk on AI but completely unable to walk the walk. I still think that's true. I think, I mean all of its talent has been leaving since long before this war and now is leaving even faster. They continue to have an educational system that churns out people that can do the theoretical side brilliantly, and their ability to apply it is weakening every year. And then it depends on where you've set the boundaries of potential adversaries. They're the two that everybody's comfortable talking about, I think, because it's quite clear.
Harry Kemsley: Sure.
Keith Dear: So yeah, hopefully that answers the question.
Harry Kemsley: What about, just briefly, what about the non- state armed groups that are out there that are frankly increasingly sophisticated in a technology sense? We've seen haven't we, the emergence of groups that are able to do pretty amazing things in the information domain, frankly, and I believe that technology is something that is spreading, it's diffused into the community increasingly. Should we not be also concerned about not just the state, but also the non- state groups that might want to use these kind of technologies against us, against our societies?
Keith Dear: Again, short answer is yes, longer answer is yes but. So I don't know if you saw the paper that was allegedly leaked from Google arguing that" We Have No Moat" right? And the reason for that is because there are increasing proliferation of open source large language models that they're slightly smaller, but their performance is practically comparable even if on some benchmarks not as good. So there's no way, I don't think, to stop the continued proliferation through the open source movement so everybody will have access. The question is, what are the boundaries on what large language models can do? We're not going to answer that here. Will non- state groups have access? Absolutely they will. And will it make their planning more efficient and effective? Yes, I think so. It might also make their execution, likewise, their ability to learn lessons, likewise, but let's not forget that they face all the same adoption challenges that states face too.
Harry Kemsley: Sure.
Keith Dear: So I don't think it's straightforward and there are still advantages to those that have access to the scale of compute that you need. And that is also a barrier where we have a potential to restrict access. I'm not absolutely, but we can limit what a inaudible access can do, I think.
Harry Kemsley: Okay. Sean, any final thoughts before we go to summarize and wrap up?
Sean Corbett: Yeah, just on the adversary side of things, I think once again we may be back to psychology. In terms of Russia, I completely agree with Keith that they're nowhere near there yet. But say Putin did have some really strong AI capability, as an autocratic despot, which he is, would he be willing to abdicate, you might say, decision making to an AI perspective? Now I think the answer to that is probably no because he, you know, unaware of those consequences. But you could say, well he almost does that now because he just believes certain people and then he acts accordingly. But for example, fast forward five years and he's got some capability and he's still fighting and being atrited heavily within Eastern Ukraine. The algorithm might say, right, your only answer is tactical nuclear weapons in terms of a pure win win on the battlefield. But the political and strategic global implication of that are huge.
Harry Kemsley: Yeah.
Sean Corbett: Would he make a rational decision on that? So I think as always, everything comes down to the human in the loop in terms of ultimately human beings are what decides up until they aren't. And this may be another podcast-
Harry Kemsley: inaudible.
Sean Corbett: ...because at what stage do we lose control? And we just go, because we can't handle that just massive amount of data do we go, " Okay, we give up." The recourse of that are huge. But coming back to our part of it, we have to follow an ethical model with AI so we don't get into that situation where individuals have too much power and authority.
Harry Kemsley: The idea that we're morally obligated based on the logical position that you've quite eloquently described, for me is quite compelling. But that argument must have been had. I mean you've been in government and in around the government bodies in the UK. Is that not enough? Is that argument not strong enough to actually convince people that we really need to be making moves here?
Sean Corbett: No, it's nothing like enough. I mean, in part because people will give examples like the one you gave Harry, right? The soldier had said, " Well what would've happened if the machine had delegated authority?" I'm like, well, what would've happened in a thousand other examples of precisely what has just been described? And if we can run that and in testing and training or in simulations, and then it should be compelling. And I know you'll get the flow of logic from the example that you gave, but not everybody does. Most people stop with the exception that says, " Hang on, what about when?"
Harry Kemsley: Yeah.
Sean Corbett: Don't point to all the many examples. You remember the inaudible incident? That was another one I was going to bring up.
Harry Kemsley: Yeah.
Sean Corbett: So the findings of that are public and they found that all the information there was there to make better decisions, but the guy was just overwhelmed, which is not really a surprise and not picking out the information that he needed. So I think, look, we can find multiple examples of where we would've made better decisions in the real world. These don't just have to be hypotheticals.
Harry Kemsley: This feels, this again, the parallels for me are quite stark, Sean, to the conversations we had in the past about the ability for people to understand what's available to the open source lends itself to needing to do it, but why aren't they? And often the argument we've come to in the past, Keith, is the lack of data literacy.
Keith Dear: Yeah.
Harry Kemsley: Knowing how to deal with data, how to understand data in the sense of its flaws, its advantage is actually one of the biggest gaps in the adoption of open source and other sources of intelligence that we just don't know how to deal with the data. We're not teaching kids at school are we data literacy per se? We're not, anymore that we teach them leadership.
Sean Corbett: That I think is as much to do with, it might be a generation thing. So the senior decision makers are the ones that aren't data literate. But I go back to, and I accept when I said never in terms of, but I know the intelligence community so well and just changing happy to glad takes about three months. So dealing with that complexity as an analyst where you know, you have to explain how you've done stuff, that they're just going to be reticent. I mean, up until the point we go, " Well this is so much more efficient, it will help you." Again, it's about the data management.
Harry Kemsley: Well either that, Sean, either that, or as Keith's point earlier, there'll be a war or some sort of conflict where frankly the rate of change is almost faster because almost always faster because it has to be, and the three months to change a word will suddenly become seconds because it won't make no sense not to. So I'm sure there'll be imperatives here, yeah. All right, well Keith, thank you for what has been, as I expected it to be, a fascinating conversation. As ever with this podcast, I'm going to ask you to give the listener a single takeaway, a final thought, what's the one thing you want the listener to take away from this conversation in AI? Unusually, I'll let Sean go after you, Keith, because I often end up eating his sandwiches as he says, and then I'll finish off. But Keith, what's the one thing you'd like the audience listening to this, the listener, as takeaway with regard to this conversation we've had, great conversation we've had about AI?
Keith Dear: I think the speed of progress. So not assuming that where we are today is where we'll be tomorrow, and then linked to that is the moral obligation to adopt various different AI models when the evidence shows it can outperform us.
Harry Kemsley: Yeah, yeah, Sean?
Sean Corbett: So I think it's all about that we have no choice regardless of what our personal view is to but to adopt, develop, and embrace AI and the debate then comes as a critical enabler or to trust it and be agile enough that when it's appropriate, let it do its thing. But also for me, and you will never say that, there has to be, at some stage, a human being that makes a decision on how much to use that information and not abdicate our responsibilities.
Harry Kemsley: Yeah, I think for me, Keith, of all the things you've said in this episode, the one that will stay with me is the phrase moral obligation, based logically on the number of false positives that AI can predictably produce versus the human. And then associated with that is that imposter syndrome mirror we're going to put up in front of the decision maker that helps them understand just how flawed they are as decision makers. So for me, the moral obligation piece is something I would like to dig further into because for me, that challenges the lack of adoption, the lack of widespread adoption. It's really saying a bit like you've said, Sean, with open source, it is almost negligent that you're not using the open source potential to the extent you should and could be, and therefore you must. Well, you've kind of said that, Keith, in a slightly different way about the moral obligation of the lower force positives we can predict with the use of AI. So for me, that's the takeaway for today. Let me finish though, as I started with a huge, huge thank you for what I knew would be, and indeed was a great conversation. It's almost every podcast episode that I say this at the end of it, we will ask you to come back and revisit some of that. I think probably the areas I'd like to dig in further for the future though would be what are we learning about the acceleration of AI and what is that doing to the arguments for adoption? For me, that's an area I'd like to dig into further. Keith, thank you so much for taking the time to speak with us today and for the listener to hear what I think has been a fantastic podcast. Thank you.
Keith Dear: Harry. Sean, thanks so much for having me. It's been great fun.
Harry Kemsley: Good stuff, Sean. Thanks as ever. We'll speak again soon. Look forward to speaking to you. And the next episode of this, we'll look, I think, to start looking in some of the more contemporary issues we're seeing about how OSINT is being used in anger against some of the issues we're seeing in the big wide world. Until then, thank you for listening and thank you again, Keith, for joining us. Thank you. Goodbye.
Audio: Thanks for joining us this week on The World of Intelligence. Make sure to visit our website, janes. com/ podcast, where you can subscribe to the show on Apple Podcasts, Spotify, or Google Podcasts so you'll never miss an episode.
DESCRIPTION
Keith Dear, Managing Director of Fujitsu's Centre for Cognitive and Advanced Technologies, joins Harry Kemsley and Sean Corbett to discuss the use and limitations of AI in support of OSINT. With AI capabilities evolving at an ever increasing speed, they explore what this means for decision makers and analysts and how human and AI can work together.
Today's Host
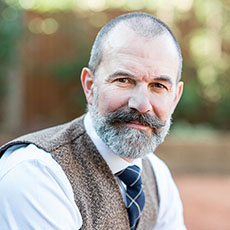
Harry Kemsley
Today's Guests
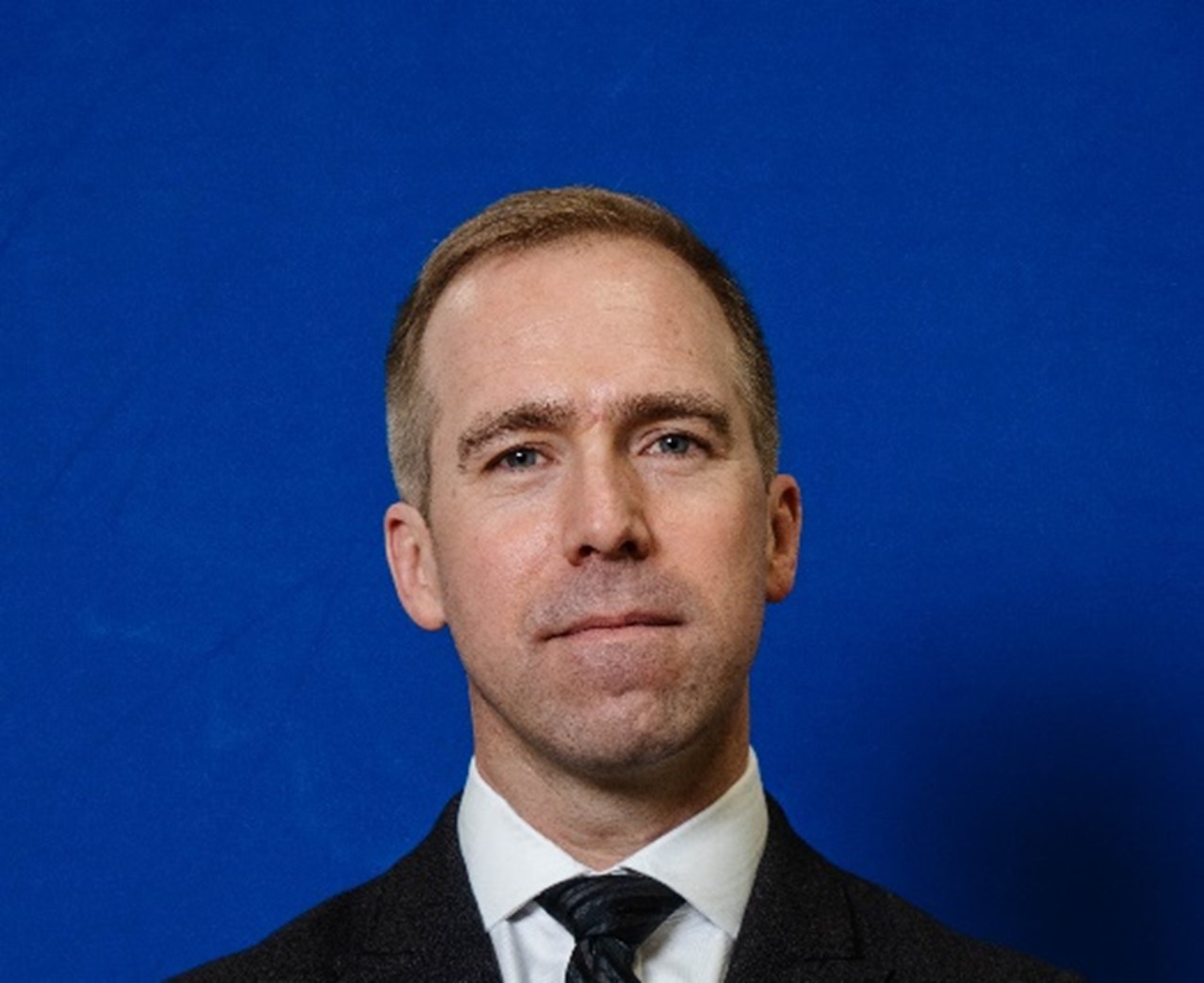